Four IEEE RA-L papers in the last year
At AIRO we published four IEEE RA-L papers in the last 12 months! While IEEE RA-L doesn’t have the highest impact factor, it is a well-received journal in the robotics and automation community. The publication of four papers in RA-L is a confirmation of our research efforts in the domain of robotics and AI.
UnfoldIR: Tactile Robotic Unfolding of Cloth (June, 2023)
Robotic unfolding of cloth is challenging due to the wide range of textile materials and their ability to deform in unpredictable ways. Previous work has focused almost exclusively on visual feedback to solve this task. In this RA-L paper we present UnfoldIR (“unfolder”), a dual-arm robotic system relying on infrared (IR) tactile sensing and cloth manipulation heuristics to achieve in-air unfolding of randomly crumpled rectangular textiles by means of edge tracing.
Compliant Robust Control for Robotic Insertion of Soft Bodies (April, 2024)
This IEEE RA-L paper proposes a novel framework for insertion-type tasks with soft bodies, such as cleaning a bottle with a soft brush. First, a multimodal model based on vision and force perception is trained. Domain randomization is used for the soft body's properties to overcome the simulation-to- reality gap. Second, we propose a dynamic safety lock method based on force perception, which is embedded in the training model to make sure that the tool explores and traverses the hole's path in a compliant way.
No More Mumbles: Enhancing Robot Intelligibility Through Speech Adaptation (May, 2024)
Spoken language interaction is at the heart of interpersonal communication, and people flexibly adapt their speech to different individuals and environments. It is surprising that robots, and by extension other digital devices, are not equipped to adapt their speech and instead rely on fixed speech parameters, which often hinder comprehension by the user. In this work we conducted a speech comprehension study involving 39 participants who were exposed to different environmental and contextual conditions.
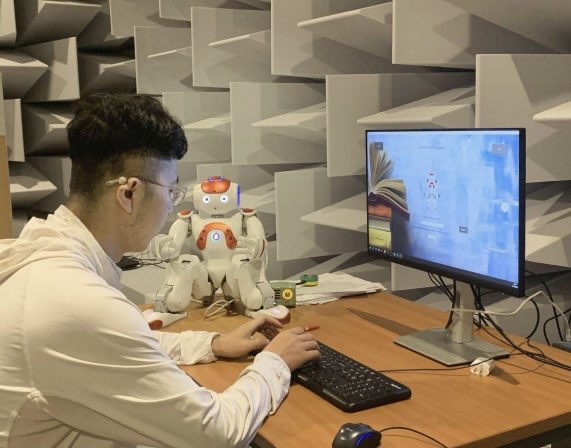
Learning Keypoints for Robotic Cloth Manipulation using Synthetic Data (May, 2024)
Assistive robots should be able to wash, fold or iron clothes. However, due to the variety, deformability and self-occlusions of clothes, creating robot systems for cloth manipulation is challenging. Synthetic data is a promising direction to improve generalization, but the sim-to-real gap limits its effectiveness. To advance the use of synthetic data for cloth manipulation tasks such as robotic folding, we present a synthetic data pipeline to train keypoint detectors for almost-flattened cloth items. To evaluate its performance, we have also collected a real-world dataset (publicly available).