PhD defence of Andreas Verleysen
Do we need to do the laundry? Or can robots help us with this task? Teaching robots to do the laundry is what Andreas Verleysen explored in his PhD which he defended today!
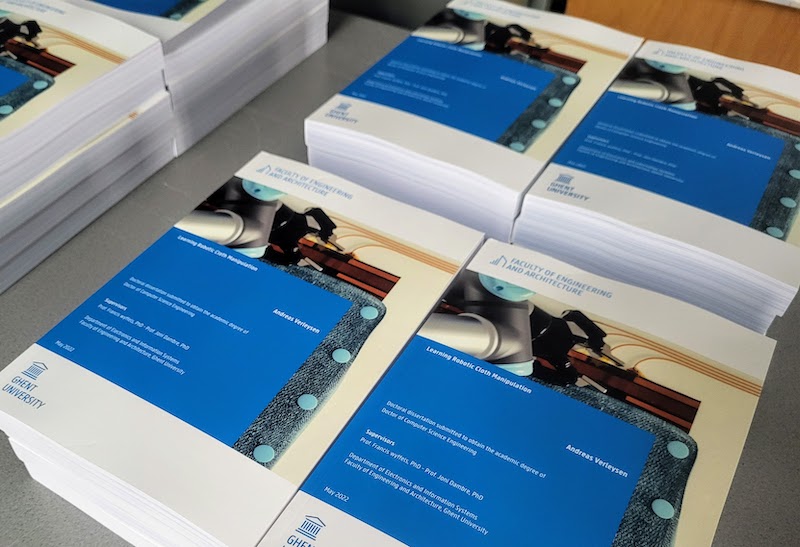
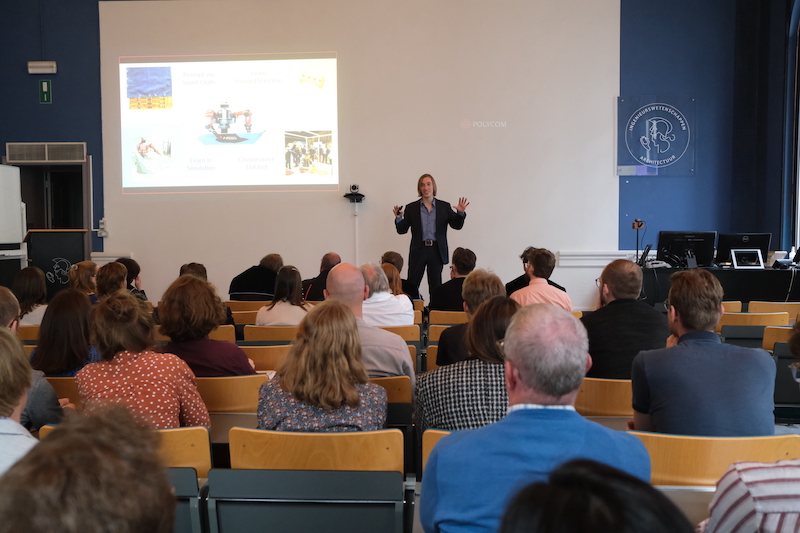
Learning Robotic Cloth Manipulation
To find methods that can handle the ever‐changing nature of human environments, we study the perception and manipulation of objects that provide an infinite amount of variations: deformable objects. A deformable object changes shape on force interaction. Deformable objects are omnipresent in industry and society: food, paper, clothes, fruit, cables and sutures, among others. In particular, we study the task of automating the folding of clothes. Folding clothes is a common household task that will potentially be performed by service robots in the future. Han‐ dling cloth is also relevant in manufacturing, where technical textile is processed, and in the fashion industry.
Dealing with the deformable nature of textiles requires fundamental improvements in both hardware and software. Mechanical engineering needs to incorporate actuators, links, joints and sensors into the limited space of a hand while using soft materials similar to the human skin. In addition to engineering more capable hands, control algorithms need to loosen assumptions about the environment in which robots operate. It is unattainable to expect highly deformable objects like cloth to always be in the same configuration before manipulating them with a robot.
A solution for dealing with real‐world variability can be found in the machine learning field. In particular, deep RL combines the function approximation capabilities of deep neural networks with the learn by trial‐and‐error formalism provided by RL. Deep RL has shown to be capable of driving cars, flying helicopters and manipulating rigid objects. However, the data requirements for training highly parameterized functions, like neural networks, are considerable. This data‐hungry property causes an incongruity between the representational learning features of deep neural networks and the high cost of generating real robotic trials.
The PhD of Andreas Verleysen focuses on reducing the required learning data for systems that perceive and manipulate clothing items. He implemented a cloth simulation method to generate synthetic data, utilized smart textiles for state estimation of cloth, crowdsourced a dataset of people folding clothing, and proposed a method to estimate how well people are folding clothing without providing labels. Learn all about the details from his dissertation.
Together with Andreas’ family, the AIRO lab members provided a nice personalised gift from poodle soup: a drawing that summarises this part of his life:
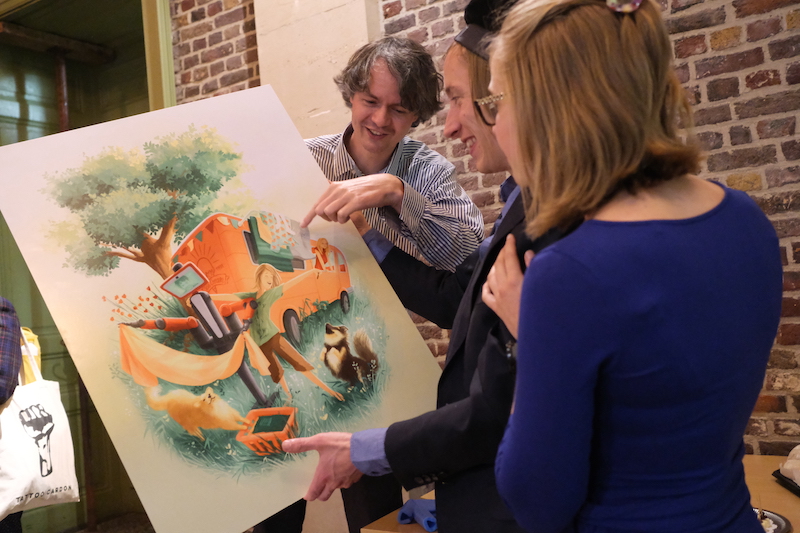
In the future, Andreas will focus on the valorisation of the learning and hardware technologies for collaborative robots here at IDLab-AIRO!